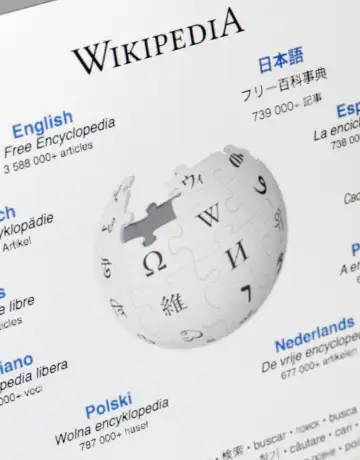
Towards Proactive Information Retrieval in Noisy Text with Wikipedia Concepts
Type
Tabish Ahmed, Sahan Bulathwela
The informational needs of people are highly contextual and can depend on many different factors such as their current knowledge state, interests and goals [1, 2, 3]. However, an effective information retrieval companion should minimise the human effort required in i) expressing a human information need and ii) navigating a lengthy result set. Using topical representations of the user history (e.g. [4]) can immensely help formulating zero shot queries and refining short user queries that enable proactive information retrieval (IR). While the world has digital textual information in abundance, it can often be noisy (e.g. extracted through Automatic Speech Recognition (ASR), PDF text extraction etc.), leading to state-of-the-art neural models being highly sensitive to the noise producing sub-optimal results [5]. This demands denoising steps to refine both query and document representation. In this paper, we argue that Wikipedia, an openly available encyclopedia, can be a humanly intuitive knowledge base [6] that has the potential to provide the world view many noisy information Retrieval systems need.
Abstract:
Extracting useful information from the user history to clearly understand informational needs is a crucial feature of a proactive information retrieval system. Regarding understanding information and relevance, Wikipedia can provide the background knowledge that an intelligent system needs. This work explores how exploiting the context of a query using Wikipedia concepts can improve proactive information retrieval on noisy text. We formulate two models that use entity linking to associate Wikipedia topics with the relevance model. Our experiments around a podcast segment retrieval task demonstrate that there is a clear signal of relevance in Wikipedia concepts while a ranking model can improve precision by incorporating them. We also find Wikifying the background context of a query can help disambiguate the meaning of the query, further helping proactive information retrieval.
Published at the First Workshop on Proactive and Agent-Supported Information Retrieval at CIKM 2022; 2022